Keynote Speakers
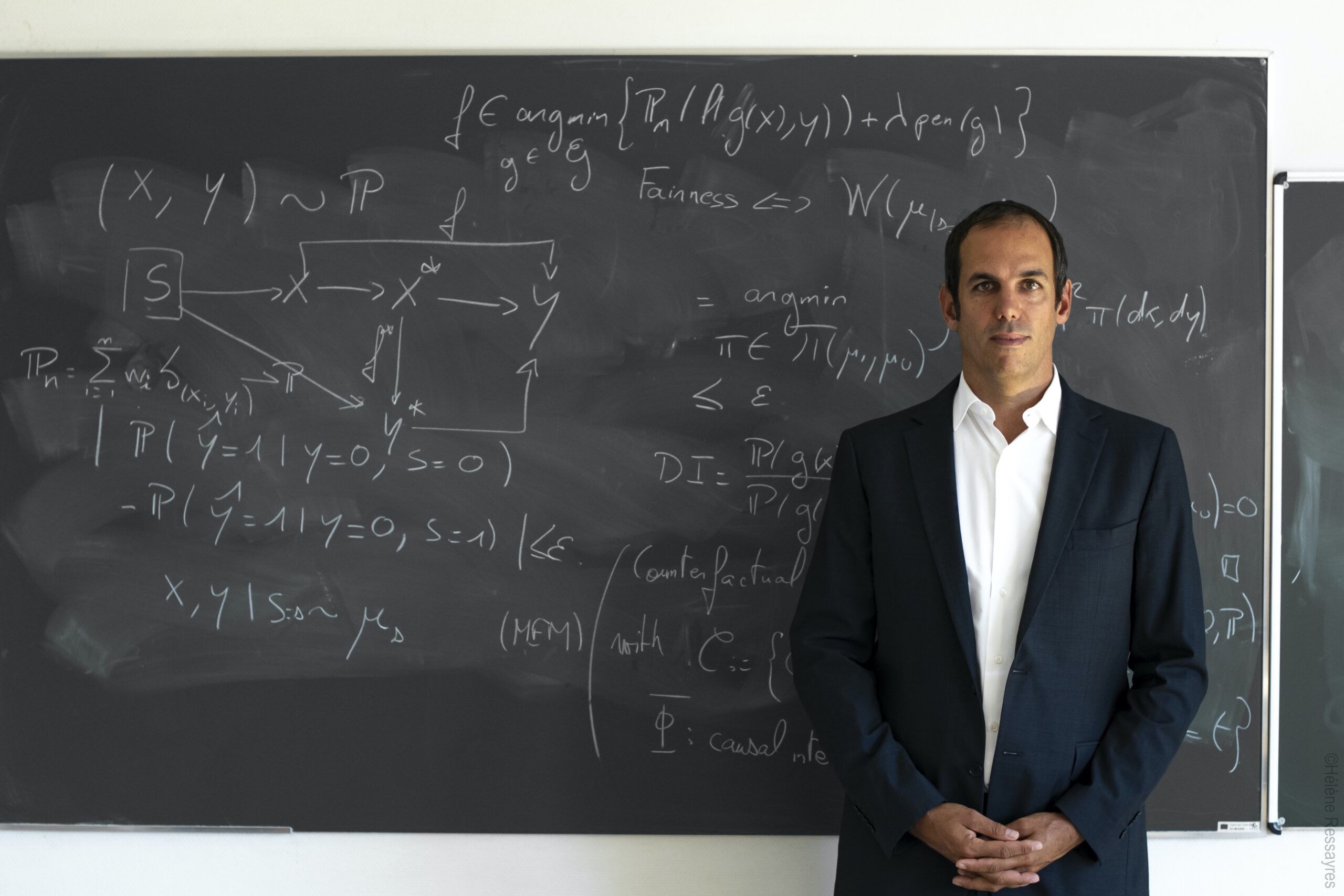
Jean-Michel Loubes
Université Toulouse Paul Sabatier, FRANCE
Jean-Michel Loubes is Professor of Statistics at the Mathematical Institute of Toulouse, University of Toulouse since 2007. Since 2019 he holds the Chair of Fair and Robust Learning at the Artificial and Natural Intelligence Toulouse’s Institute (ANITI). Since 2020, he has been elected Deputy President of the University Toulouse 3 in Charge of Innovation for Industry. He started his career as a CNRS Researcher at University of Paris Saclay and worked on asymptotic results under sparse constraints in mathematical statistics. Then his research interests turned towards developing new relevant methodological tools from probability theory and statistics to solve applications from several fields including data science, medical applications, anthropology, industrial applications and modelling of complex models. Recently, he obtained, together with his collaborators, important results in optimal transport theory and its applications to statistics and machine learning. In particular, he is interested in obtaining new methods to impose constraints to machine learning algorithms based on Monge-Kantorovich distance, which enable to reduce algorithmic biases and improve fairness of automatic decisions in Artificial Intelligence.
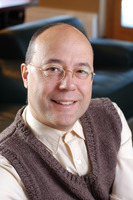
Peter Rousseeuw
Katholieke Universiteit Leuven, BELGIUM
Peter Rousseeuw has mainly contributed to robust statistics and cluster analysis. He obtained his PhD following research at ETH Zurich, which led to a book on influence functions. Later he was professor at the Delft University of Technology and at the University of Antwerp, Belgium. Afterward he spent over a decade in a financial company in New York. Currently he is professor at KU Leuven, Belgium. Among the techniques he introduced are the Least Trimmed Squares method and S-estimators for robust regression, as well as the Minimum Covariance Determinant method for covariance matrices. With L. Kaufman he coined the word medoid when proposing the k-medoids method for cluster analysis. His silhouette display for clustering is often used to select the number of clusters. Other works are the scale estimator Qn with C. Croux, robust principal component methods, regression depth, the bagplot, and detecting cellwise outliers. His 1984 paper on robust regression was included in Breakthroughs in Statistics by Kotz and Johnson, which reprinted 60 papers from 1850 to 1990.
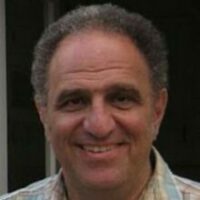
Robert Tibshirani
Stanford University, USA
Robert Tibshirani is a Professor in the Departments Health Research and Policy and Statistics at Stanford University. In his work he has made important contributions to the analysis of complex datasets, most recently in genomics and proteomics. Some of his most well-known contribution are the Lasso, which uses L1 penalization in regression and related problems, generalized additive models and Significance Analysis of Microarrays (SAM). He has co-authored over 200 papers and four widely used books. Professor Tibshirani co-authored the first study that linked cell phone usage with car accidents, a widely cited article that has played a role in the introduction of legislation that restricts the use of phones while driving. He is one of the most widely cited authors in the entire mathematical sciences field. Professor Tibshirani is a Fellow of the American Statistical Association, the Institute of Mathematical Statistics, the Royal Society of Canada (FRSC) and the Royal Society (FRS). He won the prestigious COPSS President’s award in 1996, the NSERC Steacie award in 1997 and was elected to the National Academy of Sciences in 2012.
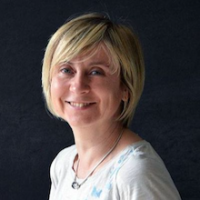
Cinzia Viroli
University of Bologna, ITALY
Professor of Statistics in the Department of Statistical Sciences, University of Bologna, since 2017. She is Associate Editor of Statistics & Computing, Statistical Methods & Applications, Journal of Classification. She is Vice President of the Scientific and Organizational Council of CLADAG (Classification and Data Analysis group of the Italian Statistical Society). Director of the international master in Statistical Sciences. Her research interests cover: Data Science, Classification and Clustering, Textual Data Analysis and Machine Learning, Latent Variable Models, Multivariate Analysis, Longitudinal and Spatiotemporal Data Analysis with application in social and economic sciences.
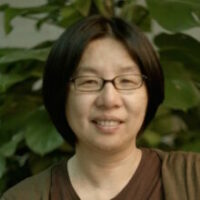
Bin Yu
University of California Berkeley, USA
Bin Yu Chancellor’s Distinguished Professor in the Departments of Statistics and EECS, and Class of 1936 Second Chair in the College of Letters and Science, both at UC Berkeley. Formally trained as a statistician, her research interests and achievements extend beyond the realm of statistics. Together with her group, her work has leveraged new computational developments to solve important scientific problems by combining novel statistical machine learning approaches with the domain expertise of my many collaborators in neuroscience, genomics and precision medicine. Yu Group also develops relevant theory to understand random forests and deep learning for insight into and guidance for practice. She is a member of the US National Academy of Sciences and Fellow of the American Academy of Arts and Sciences. She was a Guggenheim Fellow and is a former President of IMS. She serves on the editorial board of PNAS and the scientific committee of the UK’s Turing Institute for Data Science and AI.