The research objectives focus on developing statistical methods for complex data and causal inference that can be used as decision-support tools in specific domains; these tools should be interpretable, guarantee excellent predictive capabilities, and quantify uncertainty. These methods will provide comprehensive support for scientific knowledge and discovery in the Department’s domains of interest, such as demography, social sciences, economic phenomena, medicine, and epidemiology.
The main training objective concerns the strengthening of highly qualified educational programs and the training of excellent researchers. ReDS pursues the objectives of knowledge transfer to actively contribute to the innovation and technological development processes of the economic and social context of which the Department is part. To this end, ReDS also promotes professional training by promoting and stimulating interactions between DiSIA researchers and all those interested in project topics belonging to the academic and non-academic world.

Scientific objectives
Causal Analysis in Data Science
The first scientific objective of the project concerns the design and analysis of experimental and observational studies for causal analysis in light of the new challenges in data science and artificial intelligence. To answer questions of a causal nature, the ideal tool is represented by randomized experiments which cannot always be conducted: in the current era of Data Science, observational studies, including studies characterized by high-dimensional data and complex structures, represent a precious source for causal analysis, but they also pose new challenges and difficulties.
ReDS will allow us to achieve the following scientific objectives:
- Development of parametric and non-parametric Bayesian inference methods for causal analysis in experimental and observational studies with complex and high-dimensional data structures (e.g. networks, longitudinal),
- Development of conformal inference methods,
- integration of predictive machine learning algorithms with statistical methods for causal effects in high-dimensional contexts (e.g. high number of confounders),
- development of methods for data collected in the presence of (bipartite) interference, non-overlap or distribution shift,
- development of identification and estimation strategies for panel data and time series data by exploiting hypotheses about (latent) temporal and cross-sectional patterns in the data,
- development of solutions for quantifying the probability of causes and causal discovery to be used in forensic and genetic fields,
- development of new designs for online testing and new analysis methodologies will allow us to overcome the limits of A/B tests,
- development of innovative methods for the analysis of experimental studies with post-treatment complications (noncompliance, attrition etc.).
The performance and consequences of the use of machine learning algorithms will be evaluated through the development of protocols based on experimental designs and methods for risk assessment and causal inference. This will allow us to evaluate the reliability and generalizability, identifying bias, (causal) fairness problems and transparency of learning algorithms from data used as decision support in the socio-economic (e.g. personnel selection) and medical (e.g. diagnostic imaging).
Complex data integration
The second scientific objective of the project concerns the integration and modeling of complex data and the development of forecasting methods from data collected from multiple sources while respecting privacy, data sovereignty and security policies.
Specifically, the second scientific objective of ReDS consists in the development of methods for:
- big data and high-dimensional data,
- complex data (multilevel, spatio-temporal structures, networks, texts and images),
- integration of data of different types or from different sources,
- Approximate Bayesian Computation (ABC) methods,
- design of experiments and statistical models for the technological and engineering fields,
- statistical learning methods.
In this context, a major challenge concerns guaranteeing the properties of privacy, data sovereignty and data security. Furthermore, with ReDS we want to develop methods that will be clearly interpretable (interpretable AI) and computationally efficient and therefore easily applicable. The developed methodology will have direct application in many areas, with particular focus on the medical area, genomics, image data, cybersecurity, and spatio-temporal data.
Causal designs in the context of socio-demographic and economic research
ReDS aims to strengthen the role acquired by DiSIA in applied research in the demographic, social, and economic fields, by reinforcing its capacity to implement experimental designs and identify causal links within observational studies, either using censuses or sample surveys, including online surveys. Such surveys remain of fundamental importance even in the era of “big data”, addressing issues related to data quality, statistical representativeness, the lack of crucial information, and the challenge of measuring values and attitudes. However, even “traditional” surveys must adapt to the increasing complexity of socio-demographic phenomena and the related data to identify causal links.
The DiSIA-Lab will facilitate the implementation of innovative data collection designs, including online experimentation, to estimate the causal effect of marketing strategies or evaluate the impact of social and economic policy decisions on specific areas of interest. Where necessary to ensure data and results transparency and immutability over time, solutions based on Blockchain technologies will be deployed.
In particular, ReDS aims to conduct a survey on Family Complexity focusing on the causes and consequences of recent demographic transformations, with a special focus on the consequences related to education inequalities. The survey integrates ReDS’s scientific objectives, incorporating data from various sources (e.g., probabilistic and opt-in online sampling) and identifying causal links. Retrospective questionnaires will be complemented with online experiments in the form of Factorial Survey Experiments.
This survey will be conducted in close synergy with the Age-It project, an Extended Partnership on population aging (PE8), where DiSIA serves as the scientific coordinator for “The demography of ageing: A Data Science approach to decision-making” spoke. The survey complements the themes addressed by PE8, allowing DiSIA to generate innovative insights into the main socio-demographic issues facing contemporary Italy.
Steering committees
Project coordination group

Carla Rampichini
Head of DiSIA
Dipartimento di Statistica, Informatica, Applicazioni ‘G. Parenti’ (DiSIA)
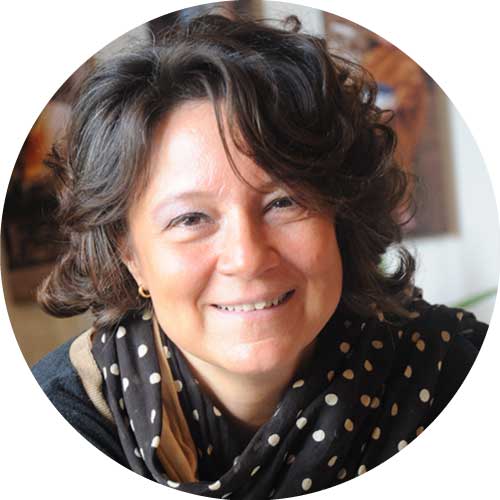
Fabrizia Mealli
European University Institute (EUI) Dipartimento di Statistica, Informatica, Applicazioni ‘G. Parenti’ (DiSIA)
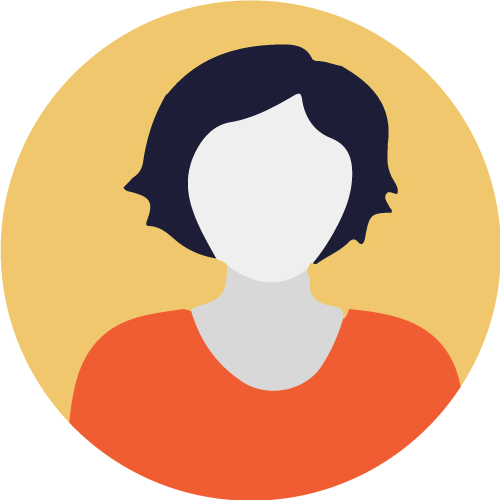
Francesca Giambona
Dipartimento di Statistica, Informatica, Applicazioni ‘G. Parenti’ (DiSIA)
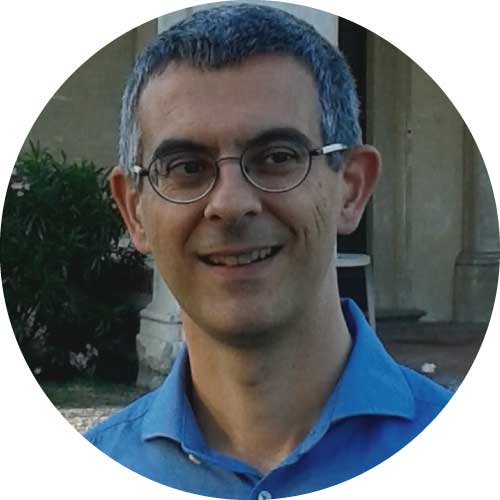
Leonardo Grilli
Dipartimento di Statistica, Informatica, Applicazioni ‘G. Parenti’ (DiSIA)
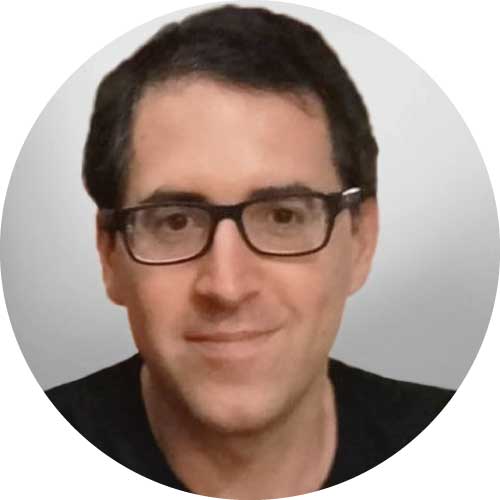
Raffaele Guetto
Dipartimento di Statistica, Informatica, Applicazioni ‘G. Parenti’ (DiSIA)
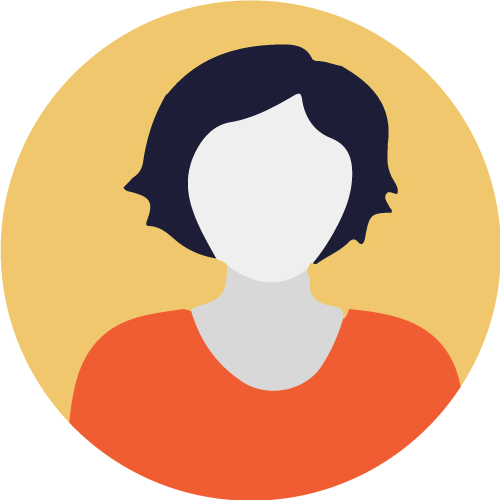
Alessandra Mattei
Dipartimento di Statistica, Informatica, Applicazioni ‘G. Parenti’ (DiSIA)
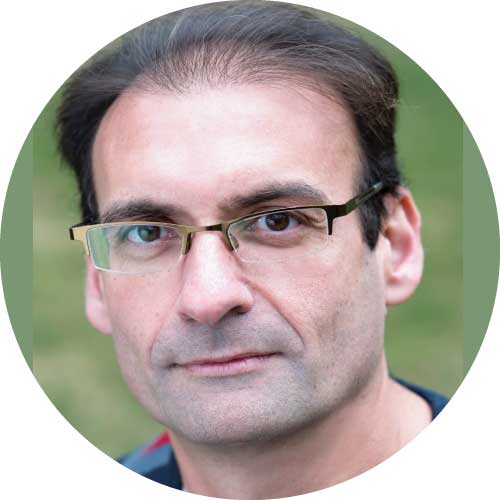
Francesco Sera
Dipartimento di Statistica, Informatica, Applicazioni ‘G. Parenti’ (DiSIA)
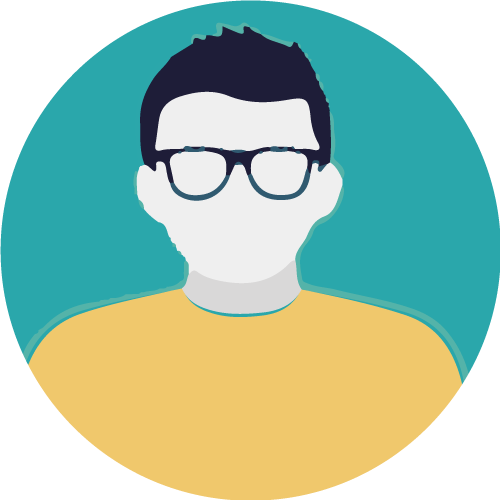
Francesco Tiezzi
Dipartimento di Statistica, Informatica, Applicazioni ‘G. Parenti’ (DiSIA)
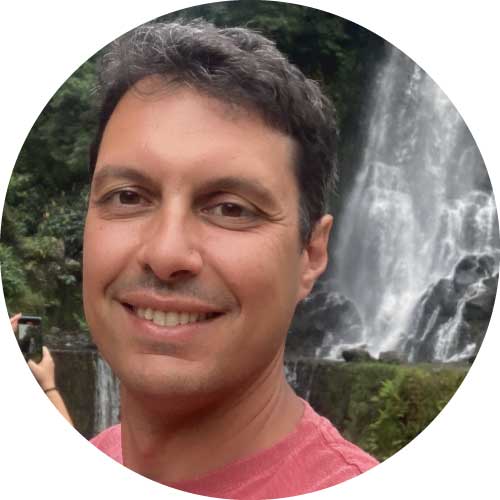
Francesco Stingo
Dipartimento di Statistica, Informatica, Applicazioni ‘G. Parenti’ (DiSIA)
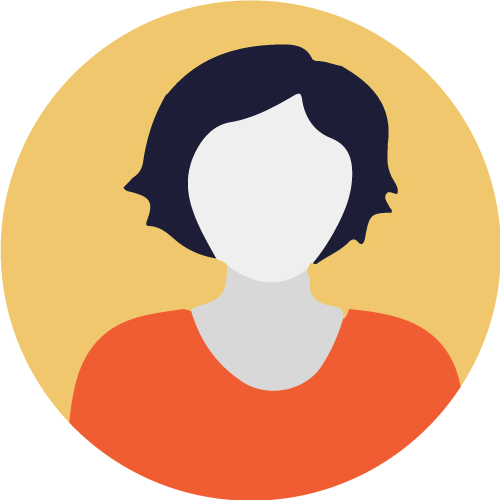
Marta Marscalchi
Dipartimento di Statistica, Informatica, Applicazioni ‘G. Parenti’ (DiSIA), research manager
Monitoring board – Graduate programs
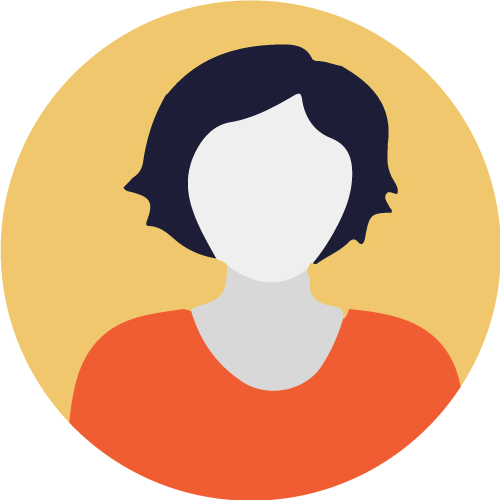
Silvia Bacci
Dipartimento di Statistica, Informatica, Applicazioni ‘G. Parenti’ (DiSIA)
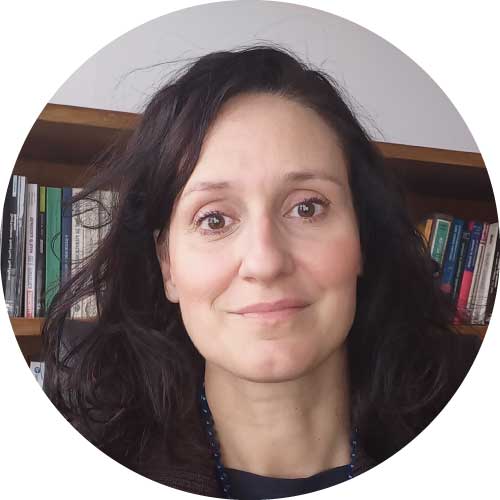
Emanuela Dreassi
Dipartimento di Statistica, Informatica, Applicazioni ‘G. Parenti’ (DiSIA)
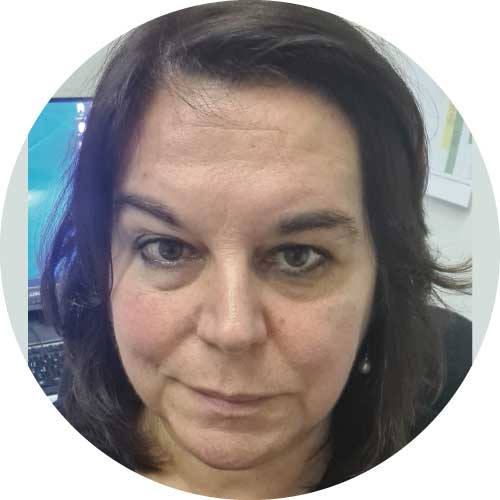
Donatella Merlini
Dipartimento di Statistica, Informatica, Applicazioni ‘G. Parenti’ (DiSIA)
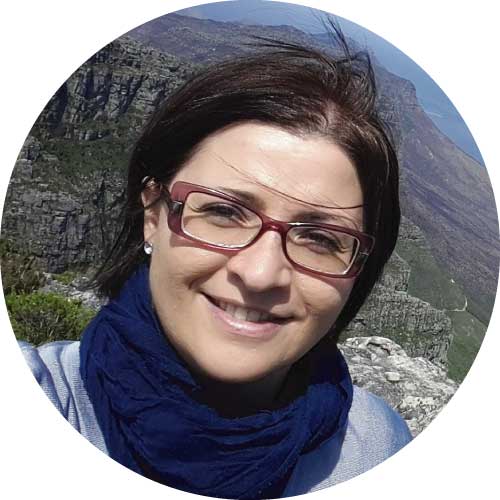
Elena Pirani
Dipartimento di Statistica, Informatica, Applicazioni ‘G. Parenti’ (DiSIA)
Monitoring board – Research
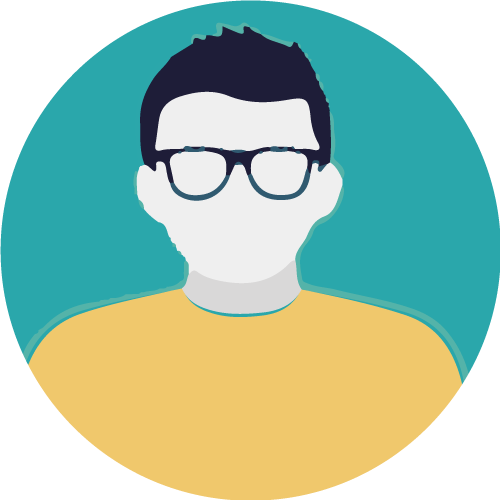
Fabrizio Cipollini
Dipartimento di Statistica, Informatica, Applicazioni ‘G. Parenti’ (DiSIA)
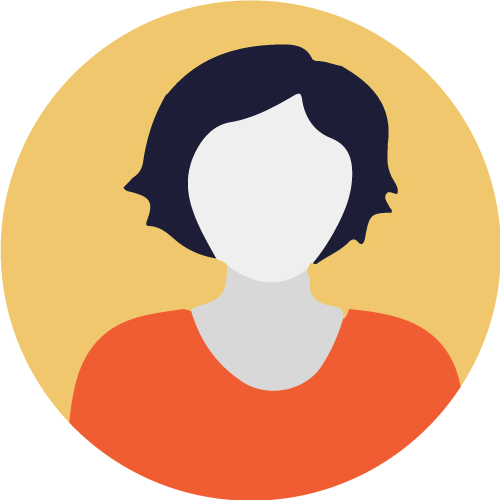
Monia Lupparelli
Dipartimento di Statistica, Informatica, Applicazioni ‘G. Parenti’ (DiSIA)
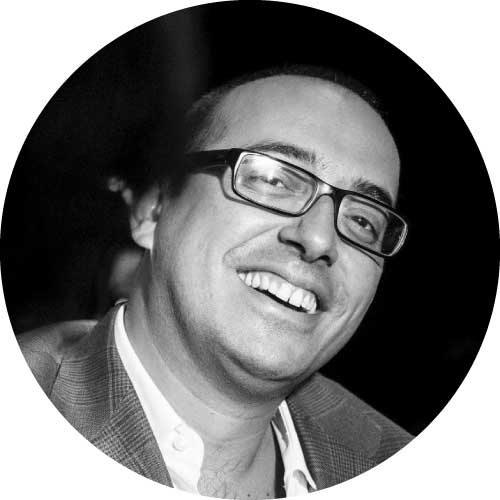
Andrea Marino
Dipartimento di Statistica, Informatica, Applicazioni ‘G. Parenti’ (DiSIA)
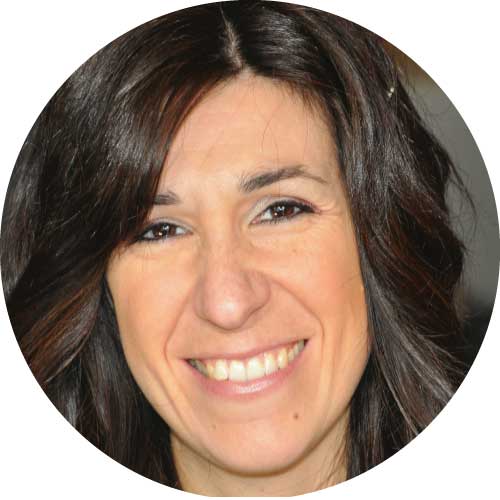
Valentina Tocchioni
Dipartimento di Statistica, Informatica, Applicazioni ‘G. Parenti’ (DiSIA)
Advisory Board
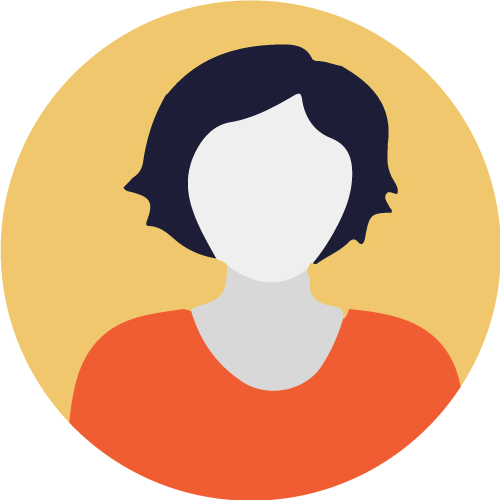
Francesca Dominici
Harvard University
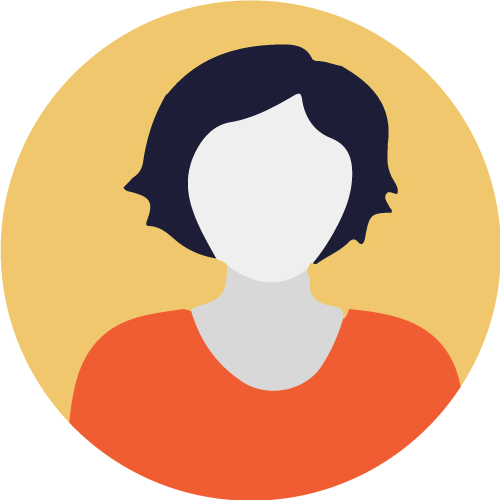
Marina Vannucci
Noah Harding Professor of Statistics, Rice University