
- This event has passed.
DiSIA Seminar
March 30, 2023 @ 12:00 - 13:00
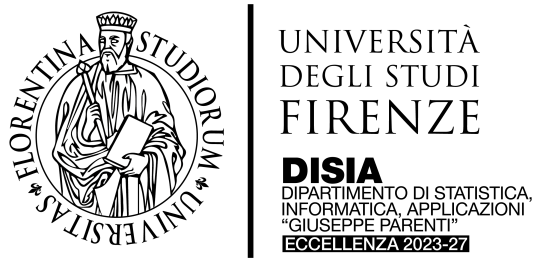
Speaker: Andrea Sottosanti (Università di Padova)
Title: Co-clustering Models for Spatial Transcriptomics
Abstract:
Spatial transcriptomics is a cutting-edge technology that, differently from traditional transcriptomic methods, allows researchers to recover the spatial organization of cells within tissues and to map where genes are expressed in space. By examining previously hidden spatial patterns of gene expression, researchers can identify distinct cell types and study the interactions between cells in different tissue regions, leading to a deeper understanding of several key biological mechanisms, such as cell-cell communication or tumour-microenvironment interaction.
During this talk, we will be presenting novel statistical tools that exploit the previously unavailable spatial information in transcriptomics to coherently group cells and genes. First, we will introduce SpaRTaCo, a new model that clusters the gene expression profiles according to a partition of the tissue. This is accomplished by performing a co-clustering, that is, a simultaneous clustering of the genes using their expression across the tissue, and of the image areas using the gene expression in the locations where the RNA is collected. Then, we will show how to use SpaRTaCo when a previous annotation of the cell types is available, incorporating biological knowledge into the statistical analysis. Last, we will discuss a new modelling solution that exploits recent advances in sparse Bayesian estimation of covariance matrices to reconstruct the spatial covariance of the data in a sparse and flexible manner, significantly reducing the computational cost of the model estimation.
By applying these tools to tissue samples processed with recent spatial transcriptomic technologies, we can gain interesting and promising biological insights. The models are in fact able to reveal the presence of specific variation patterns in some restricted areas of the tissue that cannot be directly uncovered using other methods in the literature, and, inside each image cluster, they can detect genes that carry out specific and relevant biological functions.