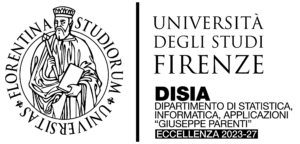
The Department of Statistics, Computer Science, Applications “G. Parenti” - DiSIA has been selected for the second consecutive time as department of excellence with a project focused on the development of methods of Causal Inference and Statistical learning. The first project "Statistics and Data Science" covered the five-year period 2018-22.
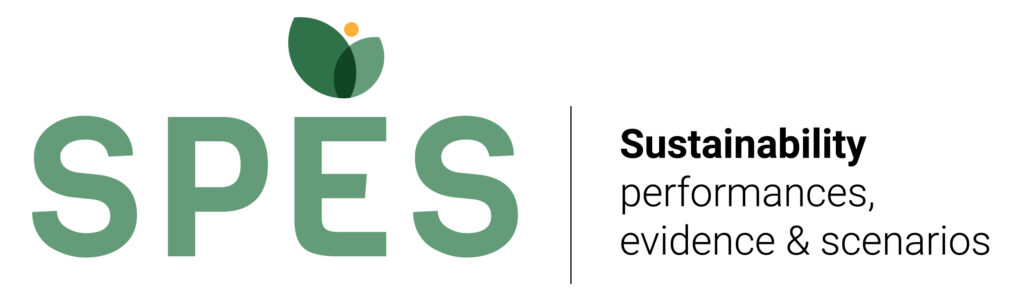
The project "SPES - Sustainability Performances, Evidence and Scenarios" is financed by the European Commission programme HORIZON-CL2-2022-TRANSFORMATIONS-01-01, for the
area called "Public policies and indicators for well-being and sustainable development". The leading partner of the project is the Department of Science for Economics and Management DiSEI of the University of Florence, which oversees the scientific coordination and operational management of all research, communication, and dissemination activities.
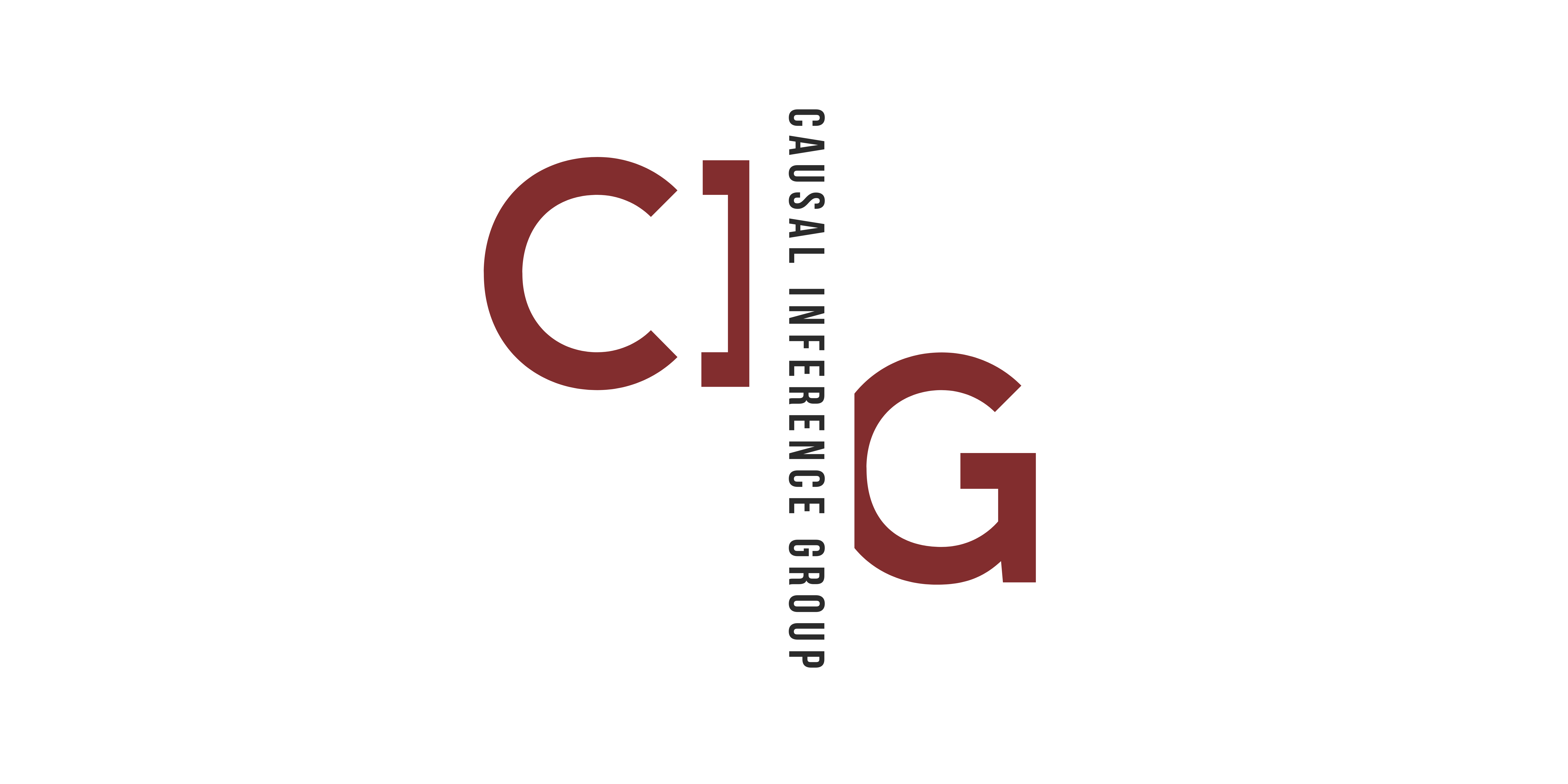
Causal Inference Group (CIG)
The Research Unit for Causal Inference comprises a dynamic and interdisciplinary team, which includes senior researchers, young scholars, and dedicated Ph.D. students. They are brought together by a shared passion for causal inference and a commitment to pushing the boundaries of knowledge in this field. Their collective efforts are aimed at addressing complex research questions, pioneering novel methodologies, and generating insights with practical applications and influence across diverse fields.
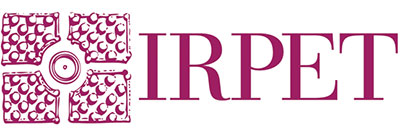
Design, management and use of statistical information systems for the analysis and forecasting of public investment projects issues and inefficiencies
Research project in colaboration with the Regional Institute for Economic Planning of Tuscany (IRPET-Istituto Regionale Programmazione Economica della Toscana)
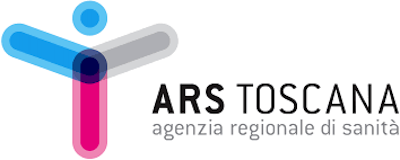
Use and developments of data science methodologies for the biomedical research
Research project in colaboration with the Tuscany Regional Health Agency (ARS-Agenzia Regionale di Sanità Toscana)
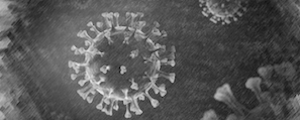
This project is intended to mobilize FDS researchers to provide data science analyses that can help to develop answers to high priority scientific questions on COVID-19.