
- This event has passed.
12th Seminar of the “D2 Seminar Series” – Florence Center for Data Science
February 25, 2022 @ 14:30 - 16:00
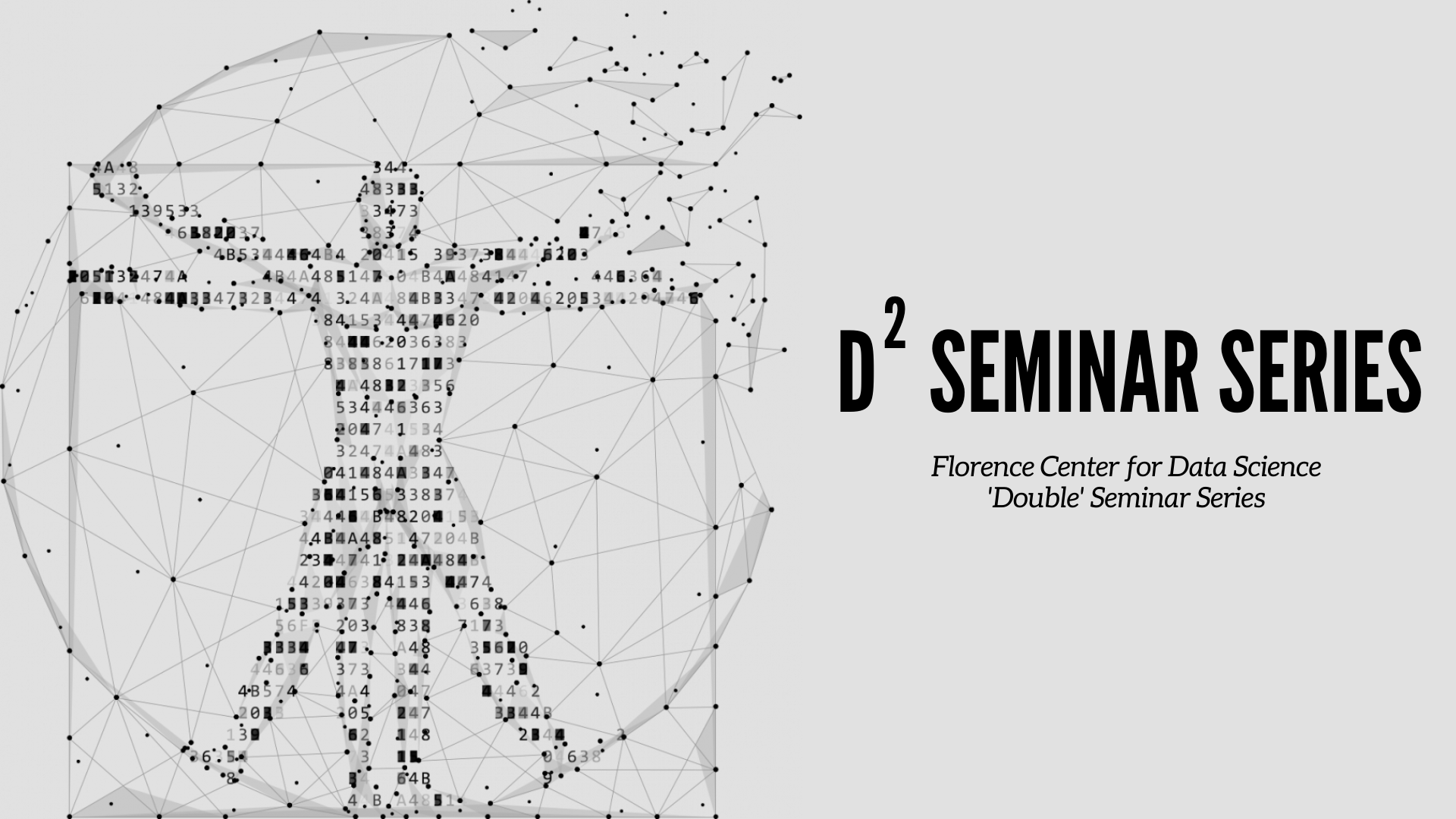
The Florence Center for Data Science is happy to present the twelfth Seminar of the “D2 Seminar Series” launched by the FDS. The Seminar will be held online Friday 25th of February 2021, from 2.30-4.00 pm.
The seminar will be held by Marco Pangallo from the Institute of Economics and of the Economics and Management in the era of Data Science (EMbeDS) of Sant’Anna School of Advanced Studies – Pisa and Fiammetta Menchetti from the Department of Statistics, Computer Science, Applications “G. Parenti” of the University of Florence.
Register in advance for this webinar:
https://us02web.zoom.us/webinar/register/WN_W9PHbIB-TQOs98cL7Z0Ilw
After registering, you will receive a confirmation email containing information about joining the webinar.
———————
Speaker: Marco Pangallo – Institute of Economics and of the Economics and Management in the era of Data Science (EMbeDS) of Sant’Anna School of Advanced Studies – Pisa
Title: Making a housing market agent-based model learnable
Abstract: Agent-Based Models (ABMs) are used in several fields to study the evolution of complex systems based on micro-level assumptions. Often, some of their micro-level variables cannot be observed in empirical data. These latent variables make it difficult to initialize an ABM in order to use it to track and forecast empirical time series. In this paper, we propose a protocol to learn the latent variables of an ABM. We show how a complex ABM can be reduced to a probabilistic model, characterized by a computationally tractable likelihood. This reduction can be abstracted into two general design principles: balance of stochasticity and data availability, and replacement of unobservable discrete choices with differentiable approximations. We showcase our protocol by applying it to an ABM of the housing market, in which agents with different incomes bid higher prices to live in high-income neighborhoods. We show that the obtained model preserves the general behavior of the ABM, and at the same time it allows the estimation of latent variables through the optimization of its likelihood. In synthetic experiments, we show that we can learn the latent variables with good accuracy, and that our estimates make out-of-sample forecasting more precise compared to alternative benchmarks. Our protocol can be seen as an alternative to black-box data assimilation methods, forcing the modeler to lay bare the assumptions of the model, think about the inferential process, and identify potential identification problems.
Speaker: Fiammetta Menchetti – Department of Statistics, Computer Science, Applications “G. Parenti”, University of Florence
Title: Combining counterfactual outcomes and ARIMA models for policy evaluation
Abstract: The Rubin Causal Model (RCM) is a framework that allows to define the causal effect of an intervention as a contrast of potential outcomes.In recent years, several methods have been developed under the RCM to estimate causal effects in time series settings. None of these makes use of ARIMA models, which are instead very common in the econometrics literature. We propose a novel approach, Causal-ARIMA (C-ARIMA), to define and estimate the causal effect of an intervention in observational time series settings under the RCM. We first formalize the assumptions enabling the definition, the estimation and the attribution of the effect to the intervention. In the empirical application, we use C-ARIMA to assess the causal effect of a permanent price reduction on supermarket sales. The Causal-ARIMA R package provides an implementation of our proposed approach.