
- This event has passed.
14th Seminar of the “D2 Seminar Series” – Florence Center for Data Science
March 25, 2022 @ 14:30 - 16:00
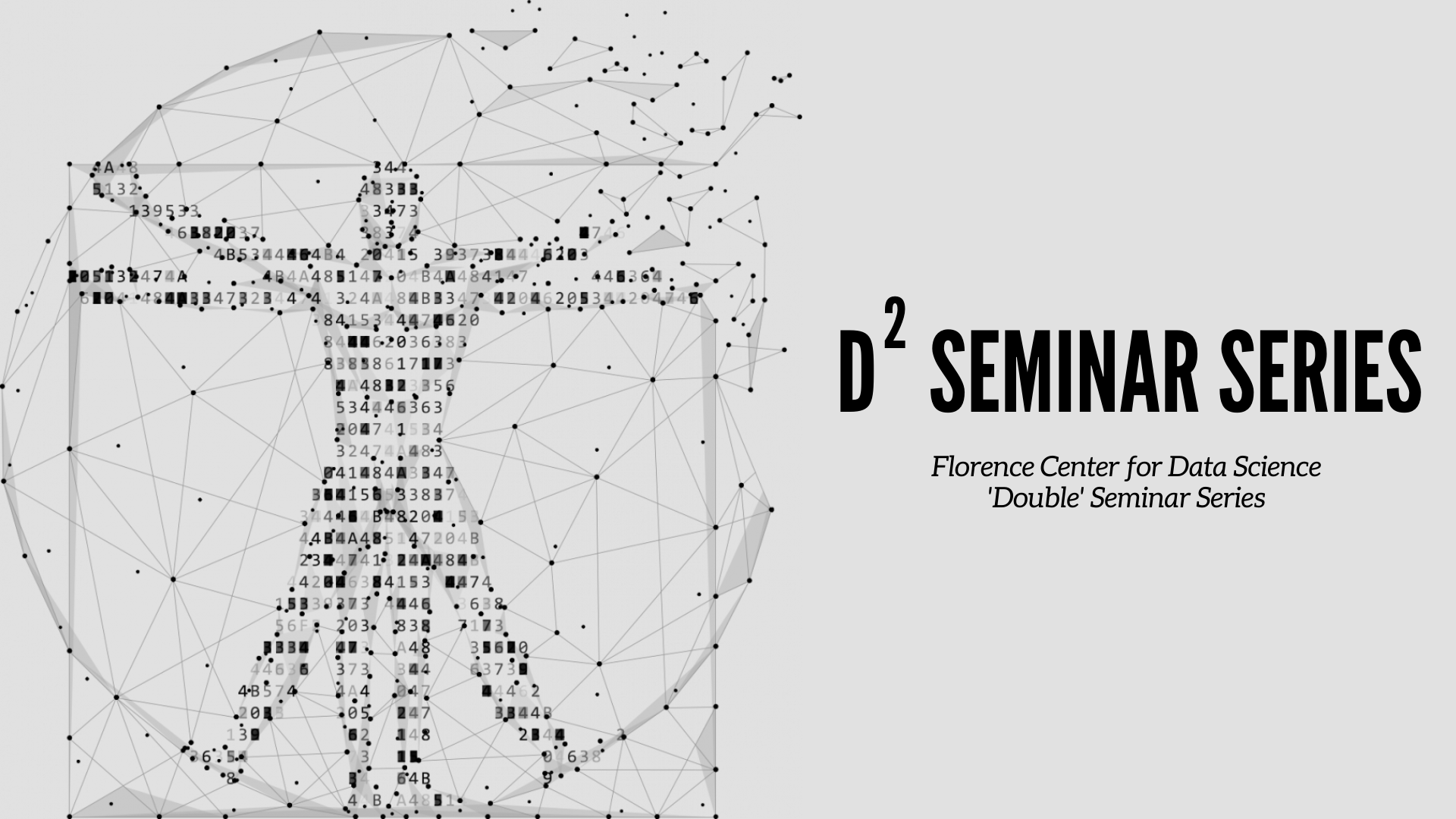
The Florence Center for Data Science is happy to present the 13th Seminar of the “D2 Seminar Series” launched by the FDS. The Seminar will be held online Friday 25th of March 2021, from 2.30-4 pm.
Brunero Liseo from the Department of Methods and Models for Economics, Territory, and Finance of the Sapienza University will present a seminar on “ABCC: Approximate Bayesian Conditional Copulae (with Clara Grazian and Luciana Dalla Valle)” and Ernesto De Vito from the Department of Mathematics of the University of Genova will present a seminar on “Understanding Neural Networks with Reproducing Kernel Banach Spaces“.
Register in advance for this webinar:
https://us02web.zoom.us/webinar/register/WN_KFoLkeSfT3-kzWLK2mwHPA
After registering, you will receive a confirmation email containing information about joining the webinar.