
- This event has passed.
DISIA Seminar: Finding the needle by modelling the haystack: pulmonary embolism in an emergency patient with cardiorespiratory manifestations
November 10, 2022 @ 12:00 - 13:00
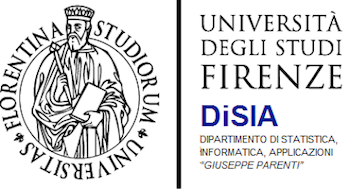
Title: Finding the needle by modelling the haystack: pulmonary embolism in an emergency patient with cardiorespiratory manifestations
Speaker: Davide Luciani (IRCCS Istituto di Ricerche Farmacologiche Mario Negri, Milano)
Location: Aula 205 (ex 32) – DISIA – Viale Morgagni 59
Abstract: A Bayesian Network (BN) was developed to perform a diagnosis covering 129 acute cardiopulmonary disorders in patients admitted to emergency departments, given an observable domain of 235 clinical, laboratory and imaging manifestations. Once the network was given a causal structure, the BN inferences could be deemed aligned to a medical reasoning framed in hundreds of pathophysiological and pathogenic related events. The structure was anticipated by experts in pneumology, cardiology and coagulations disorders, while 1,417 model parameters were estimated, via Markov chain Monte Carlo, from data of 282 records collected at the main hospital of Bergamo. The BN structure was refined until precision of diagnostic inferences improved, as long as medical literature supported any enforced structural change. Diagnostic performance was assessed by looking at the precision of predictions concerning six diagnoses, given testing findings collected from 284 records in six hospitals not including the hospital of Bergamo. Thanks to its large-size domain, the model addresses rare disorders even in patients complaining of generic symptoms. However, the size and the complexity of the model involved serious methodological challenges: to what extent causal knowledge was useful to exploit data as noisy but rich of medical information as clinical records? Was the BN causal structure faithful to the process underlying the generation of sampled data? The main lessons learned from answering these questions are introduced by taking an interdisciplinary perspective, at the intersection of knowledge engineering, evidence-based medicine, and Bayesian statistics.