
- This event has passed.
InterConnect webinar: Qiwei Li
November 10, 2023 @ 16:00 - 17:00
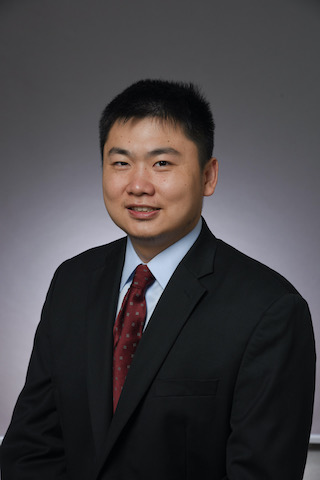
On-site and online seminar: Friday 10th November 2023 from 4.00 – 5.00 PM
Title: When statistics meets AI: Bayesian modeling of spatial biomedical data
Speaker: Qiwei Li (University of Texas at Dallas)
Location: Aula 205 (ex 32) – Viale Morgagni 59
Please register here to participate online : link
ABSTRACT
Statistics relies more on human analyses with computer aids, while AI relies more on computer algorithms with aids from humans. Nevertheless, expanding the statistics concourse at each milestone provides new avenues for AI and creates new insides in statistics. This part incubates the findings initiated from either side of statistics or AI and benefits the other. In this talk, I will demonstrate how the marriage between spatial statistics and AI leads to more explainable and predictable paths from raw spatial biomedical data to conclusions.
The first part concerns the spatial modeling of AI-reconstructed pathology images. Recent developments in deep-learning methods have enabled us to identify and classify individual cells from digital pathology images at a large scale. The randomly distributed cells can be considered from a marked point process. I will present two novel Bayesian models for characterizing spatial correlations in a multi-type spatial point pattern. The new method provides a unique perspective for understanding the role of cell-cell interactions in cancer progression, demonstrated through a lung cancer case study.
The second part concerns the spatial modeling of the emerging spatially resolved transcriptomics data. Recent technology breakthroughs in spatial molecular profiling have enabled the comprehensive molecular characterization of single cells while preserving their spatial and morphological contexts. This new bioinformatics scenario advances our understanding of molecular and cellular spatial organizations in tissues, fueling the next generation of scientific discovery. I will focus on how to integrate information from AI tools into Bayesian models to address some key questions in this field, such as spatial domain identification and gene expression reconstruction at the single-cell level.