
- This event has passed.
Seminar of the “D2 Seminar Series” – Florence Center for Data Science
October 14, 2022 @ 14:00 - 15:30
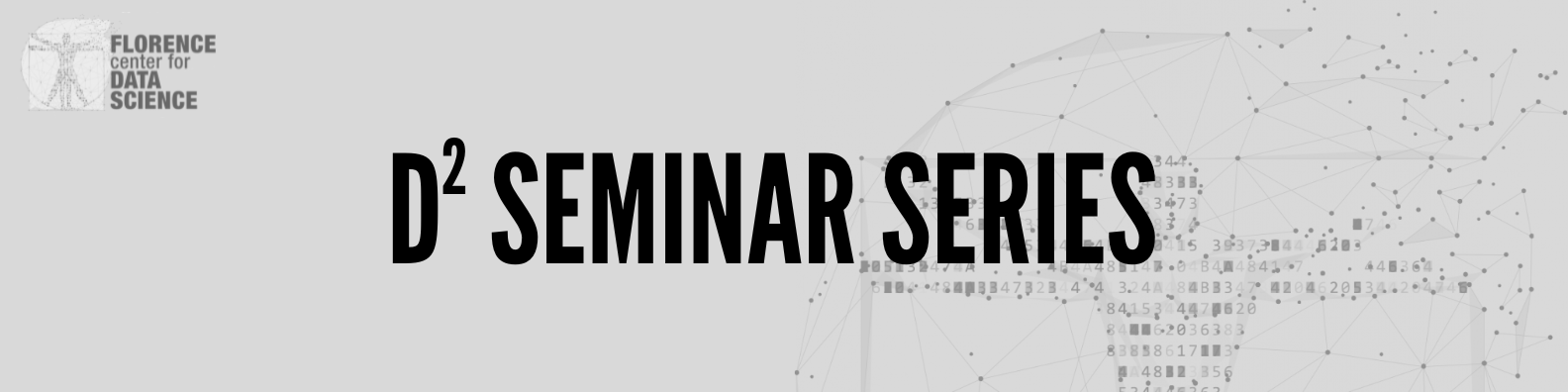
Welcome back to the new edition of the D2 Seminar Series of the Florence Center for Data Science!
We are happy to host Claudio Durastanti from the Department of Basic and Applied Sciences for Engineering (SBAI) of Sapienza University and Cecilia Viscardi from the Department of Statistics, Computer Science, Applications “G. Parenti” from the University of Florence
Claudio Durastanti will present a seminar on “Spherical Poisson Waves” and Cecilia Viscardi will present a seminar on “Likelihood-free Transport Monte Carlo“
The Seminar will be held both on-site and online Friday 14th of October 2022, from 2-3.30 pm.
The seminar will be held in Aula 205 (ex 32) (DISIA – Viale Morgagni 59).
The Seminar will be available also online. Please register here to participate online:
——-
Speaker: Claudio Durastanti from Sapienza University
Title: Spherical Poisson Waves
Abstract: During this talk, we will discuss a model of Poisson random waves defined in the sphere, to study Quantitative Central Limit Theorems when both the rate of the Poisson process (that is, the expected number of the observations sampled at a fixed time) and the energy (i.e., frequency) of the waves (eigenfunctions) diverge to infinity. We consider finite-dimensional distributions, harmonic coefficients and convergence in law in functional spaces, and we investigate carefully the interplay between the rates of divergence of eigenvalues and Poisson governing measures.
Speaker: Cecilia Viscardi from University of Florence
Title: Likelihood-free Transport Monte Carlo — Joint with Dr Dennis Prangle (University of Bristol)
Abstract: Approximate Bayesian computation (ABC) is a class of methods for drawing inferences when the likelihood function is unavailable or computationally demanding to evaluate. Importance sampling and other algorithms using sequential importance sampling steps are state-of-art methods in ABC. Most of them get samples from tempered approximate posterior distributions defined by considering a decreasing sequence of ABC tolerance thresholds. Their efficiency is sensitive to the choice of an adequate proposal distribution and/or forward kernel function. We present a novel ABC method addressing this problem by combining importance sampling steps and optimization procedures. We resort to Normalising Flows (NFs) to optimize proposal distributions over a family of densities to transport particles drawn at each step towards the next tempered target. Therefore, the combination of sampling and optimization steps allows tempered distributions to get efficiently closer to the target posterior. Finally, we show the performance of our method on examples that are a common benchmark for likelihood-free inference.