
- This event has passed.
16th Seminar of the “D2 Seminar Series” – Florence Center for Data Science
April 22, 2022 @ 14:00 - 15:30
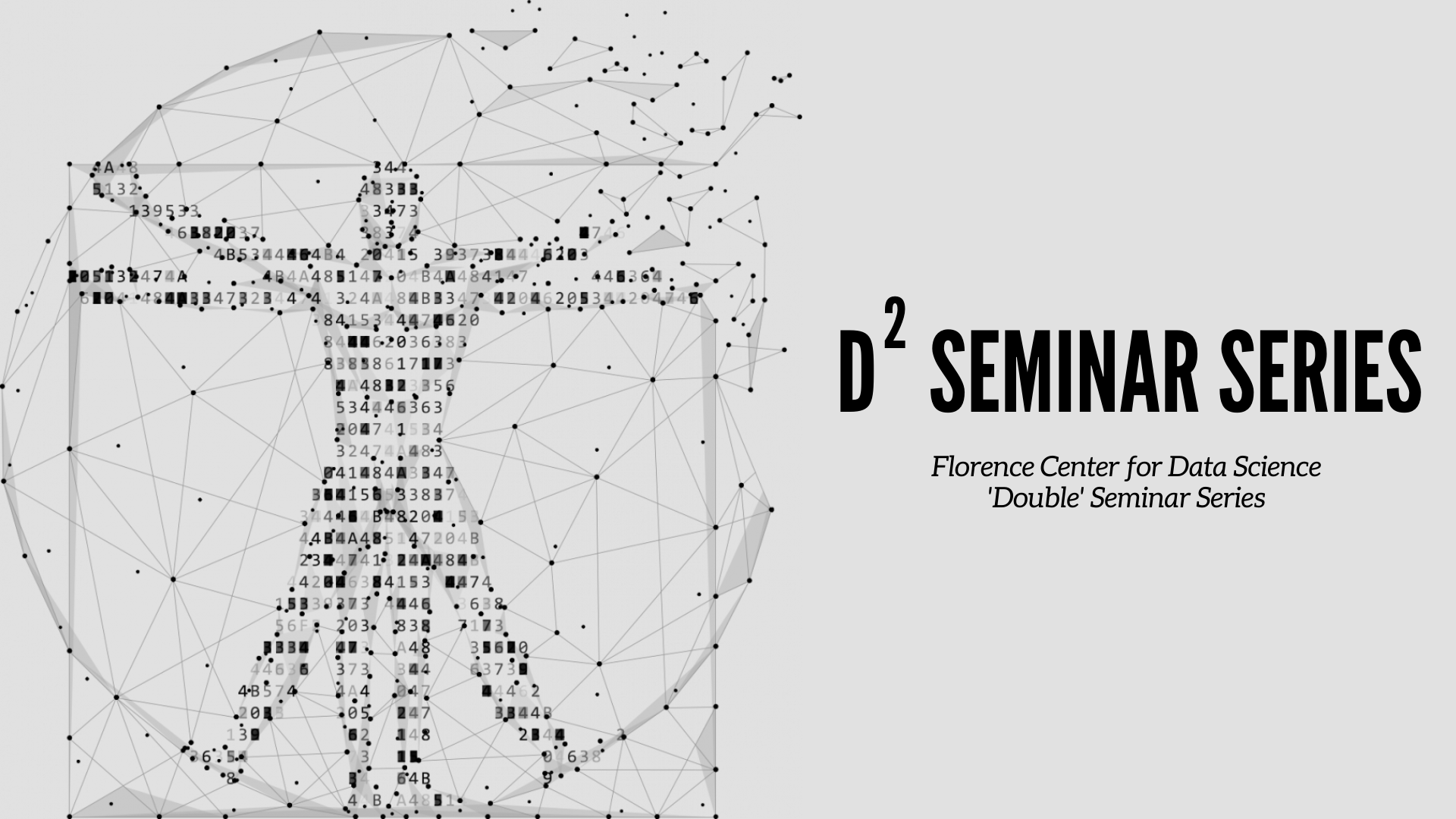
The Florence Center for Data Science is happy to present the next Seminar of the “D2 Seminar Series” launched by the FDS. The Seminar will be held on-site and online Friday 22nd of April 2022, from 2-3.30 pm.
Andrea Barucci from IFAC-CNR Institute of Applied Physics will present a seminar on “Exploring Egyptian Hieroglyphs with Convolutional Neural Networks” and Alessandra Mattei from the Department of Statistics, Computer Science, Applications “G. Parenti” of the University of Florence will present a seminar on “Selecting Subpopulations for Causal Inference in Regression Discontinuity Designs” (see abstract below).
The seminar will be held in Aula 205 (ex 32) (DISIA – Viale Morgagni 59). Participation on site is restricted and you need to register here https://labdisia.disia.unifi.it/reserve205/
The Seminar will be available also online. Please register here to participate online:
https://unifirenze.webex.com/unifirenze/j.php?RGID=rab9a115b6fac3b81f6b3fb36bb96f6da
After registering, you will receive a confirmation email containing information about joining the webinar.