
- This event has passed.
17th Seminar of the “D2 Seminar Series” – Florence Center for Data Science
May 13, 2022 @ 10:00 - 11:30
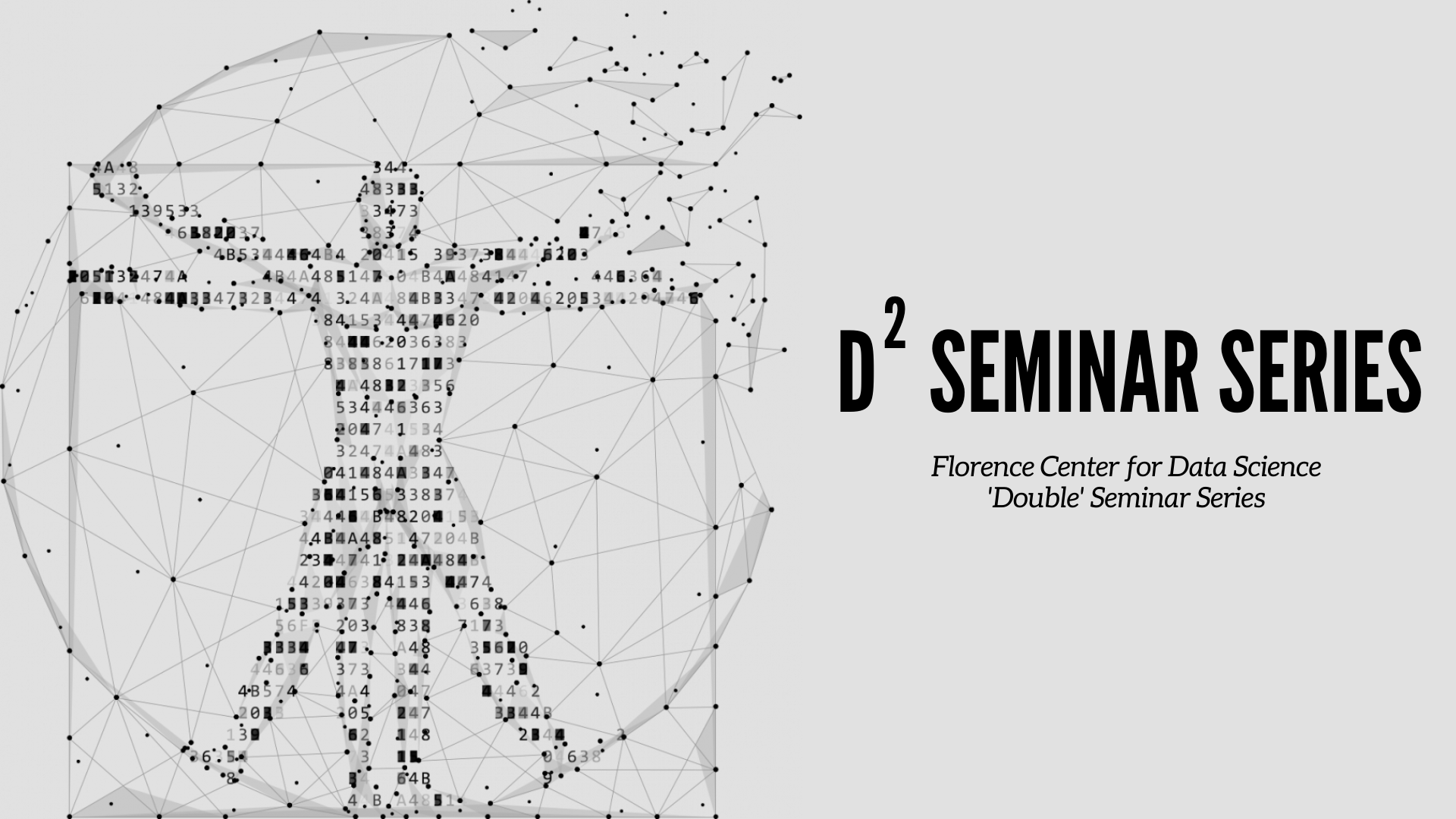
The Florence Center for Data Science is happy to present the last Seminar of the “D2 Seminar Series” for this year launched by the FDS. The Seminar will be held on-site and online Friday 13th of May 2022 from 10 to 11.30 am.
Our guests will be Georgia Papadogeorgou and Joseph Antonelli from the Department of Statistics at the University of Florida.
Speaker: Georgia Papadogeorgou – Department of Statistics, University of Florida
Title: Unmeasured spatial confounding
Abstract: Spatial confounding has different interpretations in the spatial and causal inference literature. I will begin this talk by clarifying these two interpretations. Then, seeing spatial con-founding through the causal inference lens, I discuss two approaches to account for unmeasured variables that are spatially structured when we are interested in estimating causal effects. The first approach is based on the propensity score. We introduce the distance adjusted propensity scores (DAPS) that combine spatial distance and propensity score difference of treated and control units in a single quantity. Treated units are then matched to control units if their corresponding DAPS is low. We can show that this approach is consistent, and we propose a way to choose how much matching weight should be given to unmeasured spatial variables. In the second approach, we aim to bridge the spatial and causal inference literature by estimating causal effects in the presence of unmeasured spatial variables using outcome modeling tools that are popular in spatial statistics. Motivated by the bias term of commonly-used estimators in spatial statistics, we propose an affine estimator that addresses this deficiency. I will discuss that estimation of causal parameters in the presence of unmeasured spatial confounding can only be achieved under an untestable set of assumptions. We provide one such set of assumptions that describe how the exposure and outcome of interest relate to the unmeasured variables.
Speaker: Joseph Antonelli – Department of Statistics, University of Florida
Title: Heterogeneous causal effects of neighborhood policing in New York City with staggered adoption of the policy
Abstract: In New York City, neighborhood policing was adopted at the police precinct level over the years 2015-2018, and it is of interest to both (1) evaluate the impact of the policy, and (2) understand what types of communities are most impacted by the policy, raising questions of heterogeneous treatment effects. We develop novel statistical approaches that are robust to unmeasured confounding bias to study the causal effect of policies implemented at the community level. We find that neighborhood policing decreases discretionary arrests in certain areas of the city, but has little effect on crime or racial disparities in arrest rates.